Connecting Workers with Azumuta and ChatGPT
Exponential Industry GPT reviews the connected worker platform, Azumuta. Azumuta's CEO, Batist Leman, provides feedback and insights into new levels of real-time visibility for shop floor operations.
Last week, Exponential Industry started a conversation to explore the latest in manufacturing technology with ‘Henry Elon Rogo’ a custom ChatGPT.
This week, we welcome Batist Leman, CEO of Azumuta. Azumuta is the most complete software tool that eliminates the use of paperwork in your factory. Azumuta creates connectivity across the shop floor and gets to a whole new level of real-time visibility for operations, quality control, operator training, and data collecting. Batist brings direct expertise in the connected worker space and will be critiquing the GPT responses.
GPT Becoming a Product Manager
Let’s start with an engineered prompt to put the GPT into a ‘headspace’ around connected worker technologies.
Prompt: Imagine you are a product manager for a connected worker platform product seeking to digitize manufacturing shop floor activities. Please write the prompt
As a product manager for a connected worker platform aimed at digitizing manufacturing shop floor activities, your primary focus is to develop a solution that seamlessly integrates into the workflow of manufacturing personnel, enhancing efficiency, safety, and data accuracy. Here's a prompt that encapsulates your role and objectives:
"Envision a connected worker platform that serves as a digital assistant for manufacturing shop floor personnel. This platform must be designed to streamline operations, improve communication, and support decision-making processes. Key features should include real-time data access, integration with existing manufacturing systems, and user-friendly interfaces for workers of varying technical proficiencies. The platform should support a wide range of manufacturing activities, from inventory management to quality control, ensuring compliance with industry standards. Additionally, it must prioritize worker safety by incorporating features such as hazard alerts and ergonomic recommendations. Emphasize the importance of scalability and adaptability, allowing for future technological advancements and industry shifts. Finally, consider environmental sustainability in the design, promoting energy-efficient operations and minimal waste."
This prompt captures the essence of your role, focusing on the technological, ergonomic, and sustainable aspects critical to the success of a connected worker platform in a manufacturing environment.
Batist Leman (BL): That’s a great prompt. With Azumuta we provide a solution for the aspects mentioned in the prompt: “digital assistant for manufacturing shop floor personnel”, “support decision-making processes”, “real-time data access”, “integration with existing … systems”, “user-friendly interfaces”, and so on. The “environmental sustainability in the design”, “promoting energy-efficient operations” and “minimal waste” might be a bit too generic and out of our focus, but other than that, I think I’ll make this my new elevator pitch.
Translating to Product Concepts
Since the GPT captured the essence of what we were looking for, the exact prompt was fed back in directly without edits.
The image above represents a concept for a connected worker platform in a manufacturing setting. It visualizes a digital assistant platform that integrates seamlessly with existing manufacturing systems and is designed to be user-friendly for workers with various technical backgrounds. The platform supports a range of activities, from inventory management to quality control, while ensuring compliance with industry standards. It also prioritizes worker safety through features like hazard alerts and ergonomic recommendations. Additionally, the design is scalable and adaptable, ready for future technological advancements and industry shifts, with a strong focus on environmental sustainability to promote energy-efficient operations and minimal waste.
BL: Actually, I liked the prompt more than the image and vision given here. The GPT failed to address the key factors for shopfloor solutions as mentioned in the prompt. Based on what we’re seeing in the industry:
More and more product variations and growing demand for personalized products increase the complexity for the worker. This, combined with a labor shortage and lower-skilled workers, leads to more quality problems and lower productivity if the worker is not properly supported. This necessitates a solution that has:
Context-aware digital work instructions that automatically adapt according to the specifications of the product (through ERP integration) and the competency level of the operator.
Inline quality control is integrated into the work instructions so that problems are addressed immediately and scrap can be avoided.
Competency management around who knows what, knowledge preservation, on-the-job training, finding and training of employees. It should support suggestions for up-skilling and re-skilling based on real performance.
Shop floor feedback and communication with the people on the floor. Learning from each other involves the employees.
Suggesting Features
The prompt generated by the GPT produced a good elevator pitch but the GPT struggled to address any capabilities needed for the connected worker platform. A hands-on real-world practitioner, like Batist, can clearly articulate the market needs and product requirements for a connected worker platform. Let’s see if we can have the GPT “fetch” from Azumuta’s website and suggest new features.
Prompt: Review Azumuta's platform and suggest additional features they should invest in based on the concepts envisioned.
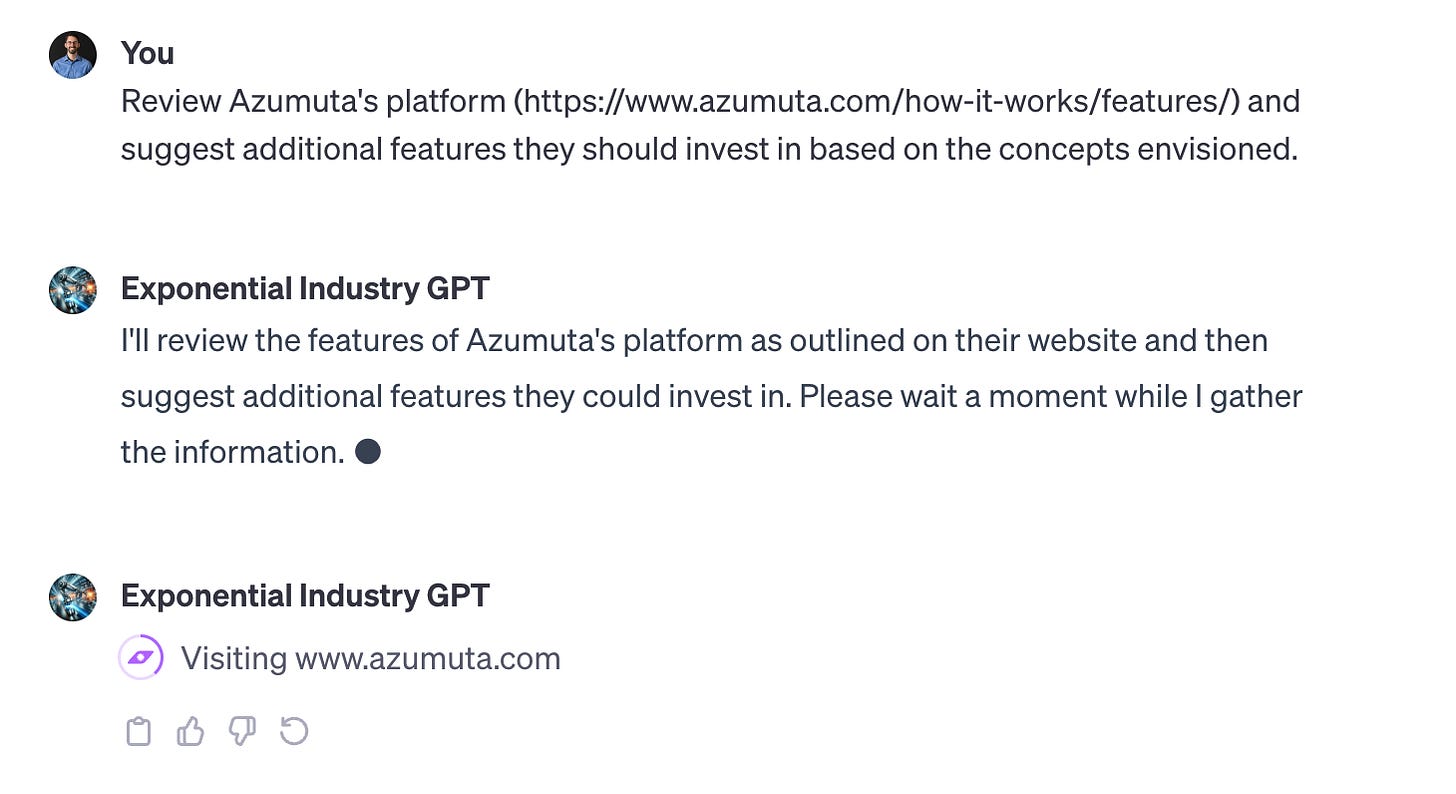
Azumuta's platform offers a range of features designed to enhance manufacturing operations:
…
Quality Management: The platform focuses on improving operational quality, reducing human errors, and enhancing quality control.
…
Based on these features, here are additional feature suggestions for Azumuta, aligning with the connected worker platform concept:
Enhanced Real-Time Communication: Implement a more robust real-time communication tool that allows for instant messaging and video conferencing among shop floor workers and management. This would facilitate quicker problem-solving and decision-making.
Integrated Wearable Technology: Incorporate compatibility with wearable devices that can monitor worker health and safety, like smart gloves or glasses. These could provide hands-free access to work instructions and real-time hazard alerts.
Environmental Sustainability Analytics: Introduce features that track and analyze the environmental impact of manufacturing processes, such as energy consumption, waste production, and carbon footprint. This would support companies in their sustainability goals.
Predictive Maintenance Tools: Develop capabilities for predictive maintenance using AI and machine learning algorithms. This would help anticipate equipment failures and schedule maintenance, thereby reducing downtime.
Customizable Dashboard and Reporting: Enhance the data analytics section with more customizable dashboards and reporting tools. This would allow different levels of management to access tailored information relevant to their specific needs.
Integration with IoT Devices: Strengthen the platform's integration capabilities with IoT devices on the shop floor, enabling better data collection and analysis for continuous improvement and operational excellence.
These suggestions are aimed at further enhancing the digitization and efficiency of shop floor activities, aligning with the evolving needs of modern manufacturing environments.
BL: GPT’s review of Azumuta’s How it Works page does include all of the basic features of Azumuta’s software. However, the GPT just rephrased what’s already written on the description of each card, such as shown below under the “Quality Management” card.
BL: The GPT did not go into detail on any of Azumuta’s modules and did not mention the complete features of each module. The GPT’s current understanding of Azumuta is still relatively shallow. The GPT suggested 6 additional features with our responses to each suggested feature with up to one point credit given to each.
Enhanced Real-Time Communication
BL: The GPT suggested real-time text and video-based two-way communication features. At the moment, we already have a text-based two-way communication feature under our Digital Work Instructions module. But it is mainly directed towards asynchronous communication.
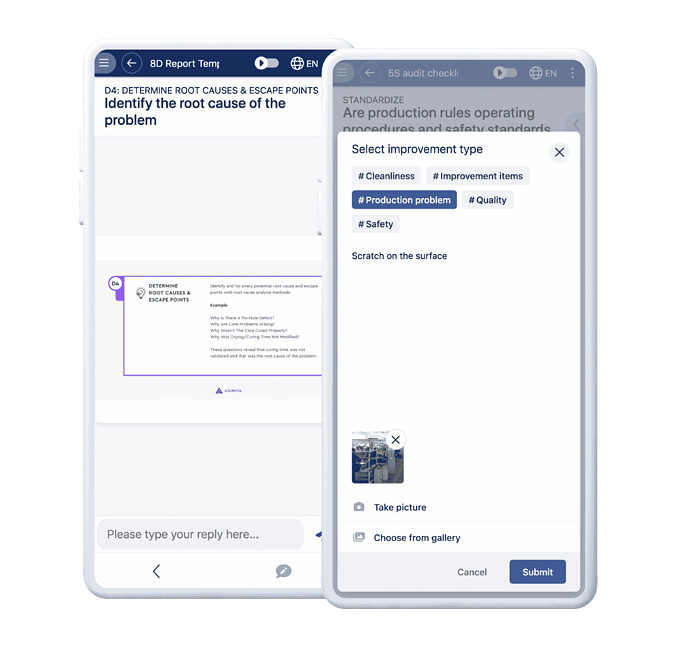
A video-based two-way communication system is actually a good suggestion, and the reason we’re partnering with SupportSquare to incorporate this functionality, so a good suggestion! And we are on it!
Credit: 1
Integrated Wearable Technology
BL: GPT recommended that Azumuta integrate wearables such as smart gloves and glasses. While we do support this functionality through a flexible API, there is a lot of “lower hanging fruit” and in our experience, most companies are focusing on first. We typically recommend our customers start walking before trying to run into complex integrations.
Credit: 0.75
Environmental Sustainability Analytics
BL: Sustainability-related measurements such as energy consumption, waste output, and carbon footprint are often measured by their own specific tools. For example, electricity consumption by the electricity vendor, carbon emissions are measured by built-in sensors, etc. Not quite sure how we can help with this.
Credit: 0
Predictive Maintenance Tools
BL: Currently, Azumuta’s software supports only preventive maintenance, although the possibility of adding a predictive maintenance feature is always open. This is indeed a relevant suggestion posed by GPT. In our experience, however, it’s often a better idea to use other systems as a driver. Systems like Augury can do a continuous monitoring of systems. Then, when maintenance is required, a trigger can be sent by Augury to Azumuta to schedule the procedure. Once the operator performs the procedure, a trigger is sent back from Azumuta to Augury. Good suggestion!
Credit: 1
Customizable Dashboard and Reporting
BL: Good to know it’s not clear from our website, but Azumuta does already have this functionality built in. All data captured by the different modules feed into our dashboards. Users can then create visually intuitive data dashboards, granting them full situational awareness of their shop floor. We also expose this data through our APIs, allowing advanced users to connect it to their data tools like PowerBI or Tableau.
Credit: 0.75
Integration with IoT Devices
BL: Again, we do already have this feature, the GPT might be hallucinating a bit here, we do use the term “peripherals” to indicate the “IoT” is connected to the workstation, that might be the reason. Our software can be connected to countless devices, ranging from simple digital scales to torque tools, to inspection cameras. Thanks for the suggestion GPT, we’re a step ahead here.
Credit: 0.5
4/6 points being helpful for a few minutes of work is not a bad ROI at all!
Stretch Tasks
Azumuta already supports IoT device integrations such as digital torque wrenches. Let’s see if the GPT can connect wrench usage as described in an integration video to predictive maintenance approaches.
Prompt: Watch "How to Integrate a Digital Torque Wrench with Digital Work Instructions" video and recommend the types of algorithms that would work well for predictive maintenance on torque wrenches.
I've accessed the YouTube video titled "How to Integrate a Digital Torque Wrench with Digital Work Instructions" from Azumuta's YouTube channel, as you requested. However, as a text-based AI model, I'm unable to watch videos. Nevertheless, I can recommend types of algorithms suitable for predictive maintenance on torque wrenches based on my existing knowledge.
Time-Series Analysis Algorithms: These algorithms, like ARIMA (AutoRegressive Integrated Moving Average), can analyze historical usage data of the torque wrench to predict future maintenance needs based on patterns and trends over time.
Machine Learning Regression Models: These models, such as linear regression, random forest, or gradient boosting, could be trained on historical data to predict when a torque wrench might fail or require maintenance, based on factors like usage frequency, torque settings, and environmental conditions.
Anomaly Detection Algorithms: Algorithms like Isolation Forest or One-Class SVM (Support Vector Machine) can be used to detect anomalies in the operational data of the torque wrench. Anomalies might indicate potential issues or the need for maintenance.
Neural Networks: Deep learning models, particularly recurrent neural networks (RNNs) or Long Short-Term Memory (LSTM) networks, could be effective in predicting maintenance needs by learning from complex and large datasets that include operational parameters and historical maintenance records.
Clustering Algorithms: Algorithms like K-means or hierarchical clustering could be used to group similar usage patterns or maintenance needs, helping to predict maintenance schedules more accurately.
Implementing these algorithms would involve collecting and analyzing data from the digital torque wrenches, such as torque applied, number of uses, duration of use, and environmental conditions. This data would then be used to train the predictive models, which can then forecast potential maintenance requirements, thereby reducing downtime and increasing the lifespan of the tools.
BL: It’s impressive to see what it suggests just from the title and question, it didn’t even need to watch the video! However, I’m sure there are better tools to do preventive maintenance on torque tools than Azumuta. As mentioned in a previous question we do facilitate other, better equipped, software tools to use Azumuta to communicate with the operator. Additionally, we can also provide some extra context to some data. For example: in what order was the operator working when an error occurred? Who was the frontline worker? This unlocks a series of extra questions that can be answered, that would otherwise not have been possible to answer, such as: Could it have affected the product? Does a torque tool error/wear affect recalls? Are some workers causing more strain on the tools than others? Is extra training on how to use the tool beneficial for the lifespan of a tool?
GPT for Manufacturing Startup CEOs
BL: Overall, the GPT helped probe my company and the product space we operate in, but it misses the real-world shop floor context. That’s why I think the Exponential Industry GPT approach is so interesting, as it will learn from articles curated with rich context about manufacturing operations and applied technology.
With that, we close out with a few questions for Batist on how generative AI and Exponential Industry is impacting Azumuta.
Question: How are you leveraging generative AI and chatbots within your company? Are you planning to include it within your platform? If so, how?
BL: Yes, absolutely. We are actively working on this and already have this in production in a couple of areas:
Creation of work instructions: We use the OpenAI to segment voice descriptions to automatically generate work instructions, significantly increasing the speed of instruction creation.
Improving the instructions: We have several AI techniques to improve the crispness and understandability of work instructions. We improve these, based on the feedback of the operators. Companies can opt-in to share their own instruction best practices, in return, the AI will suggest how to make their instructions better and will automatically propose things to change.
Instruction conversion: Companies often have existing work instructions. We provide a toolset that helps with this conversion. What used to take days can now be done in an instant.
Instruction consumption: In some cases, there can be a lot of information available and you want to pull the right info - fast. AI can help to understand the context and offer the correct information.
Adapting instructions: Not all frontline workers have the same skill level, some make more mistakes than others. Here we can automatically adapt the amounts of quality checks, based on previous quality results. Although a lot can be done with ‘basic math’, AI systems are quite good at handling extra context complexity like: “that day it was super hot outside and all tolerances were off that day, it’s not worker related”
Analytics: Although it’s not core to our product to do advanced analytics, we do provide insights into the data we are generating. LLMs are quite good with “low” amounts of data with a high rate of complexity.
An important side note is data privacy. Sometimes companies fear that their data will “leak” into the AI, as the AI learns from the questions. But that’s not the case, and OpenAI now guarantees data privacy. We also see a lot of companies setting up their systems on Azure, to have full control.
Question: What do you enjoy most about Exponential Industry?
BL: For me personally, it’s a perfect aggregation of what’s going on in the field of manufacturing. The newsletter is densely packed with information I would otherwise have to search dozens of websites for. Exponential Industry also feels very engineering-oriented, while I think other resources can sometimes feel a bit too ‘high level’ and marketing speak.
Continue the Exponential Industry GPT series…